A new EU Horizon project has just been confirmed, named WeatherGenerator, which aims to use artificial intelligence in novel ways for weather forecasting and for modeling related Earth system processes.
The WeatherGenerator is being developed by 16 European organizations (list below), in a four-year EU-funded initiative which is to start in February 2025. The initiative is led by ECMWF while the participants include leading national meteorological services, research centers and industry partners active in high-performance computing, machine learning and applications.
- ECMWF (International)
- Max-Planck-Gesellschaft zur Förderung der Wissenschaften e.V. (Germany)
- Forschungszentrum Jülich (Germany)
- Norwegian Meteorological Institute (Norway)
- Royal Netherlands Meteorological Institute (Netherlands)
- Météo-France (France)
- Swedish Meteorological and Hydrological Institute (Sweden)
- UK MetOffice (UK)
- Centro Euro-Mediterraneo sui Cambiamenti Climatici (Italy)
- Netherlands eScience Center (Netherlands)
- Buluttan Weather Intelligence (Turkey)
- Latest Thinking (Germany)
- Kajo Services (Slovakia)
- Statkraft (Norway)
- ETH - Eidgenössische Technische Hochschule (Switzerland)
- MeteoSwiss (Switzerland)
Weather Generator in a Nutshell
The fundamental idea behind WeatherGenerator is to build one earth system model that can be used and adapted for a large number of specific tasks. That is why the WeatherGenerator can be regarded as a foundational model rather than a task-specific machine learning model.
WeatherGenerator project stands out for its comprehensive approach to weather and climate modeling. Unlike typical task based AI-based weather models, it is designed to be a flexible, general-purpose tool that can handle a wide range of input data sources, including reanalysis datasets, meteorological & land surface observations and outputs of conventional models. This allows the WeatherGenerator to perform a range of tasks like forecasting, data-assimilation, downscaling, post-processing, making it a versatile model for a multitude of weather and climate applications.
The conceptual idea of the WeatherGenerator is to let all the data streams come in and to then learn the statistical correlation across the space/time dimensions so that one can be transformed into another.
In comparison, other AI-based models like NVIDIA’s FourCastNet, Huawei’s PanguWeather, and Google DeepMind’s GraphCast are more task-based specialized models. FourCastNet uses neural operators to naturally handle spherical geometry and focuses on medium-range forecasts, offering high stability and spatial resolution. Similarly, PanguWeather excels at rapid global predictions using a neural network model. GraphCast leverages Graph Neural Networks (GNNs) to efficiently model spatial relationships and produces skillful 10-day forecasts. As a summary, WeatherGenerator provides a highly adaptable framework that can be applied across many domains, from research to operational forecasting, whereas models like PanguWeather, GraphCast, and FourCastNet excel at specific forecasting tasks with a focus on accuracy, speed, and medium-range predictions.
Under The Hood
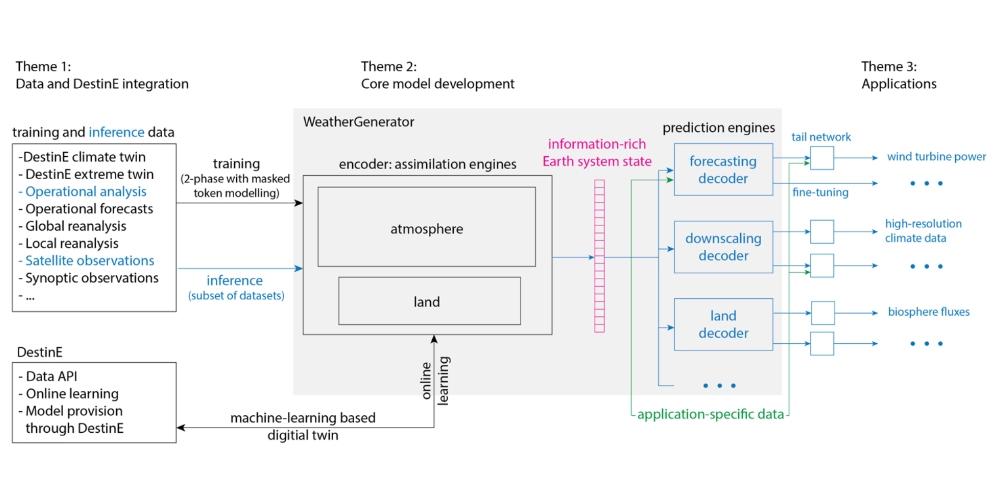
An encoder-decoder neural network architecture is at the core of the WeatherGenerator design. The encoder component is acting as an ingestion engine that combines many different data sources such as global reanalyses, local area models (LAMs) and observational data. It then encapsulates this information into a rich Earth System State that is independent of any grid & resolution.
During the operational execution of this architecture, the encoder requires only a subset of the inputs used for training. This is expected, as not all data inputs will be available during inference. The decoder will then act as a prediction engine performing auto-regressive forecasting, downscaling, assimilation or nudging. For this, it will rely on the rich representation from the encoder, potentially complemented by task-specific data inputs such as high-resolution orography or application specific data.
The approach taken to train a machine learning tool that can handle a large number of different inputs is called masked token learning. The idea is similar to the Masked Self-Attention concept where LLMs learn rich relationships and patterns between the words of a sentence.
In this approach, parts of the data from the input streams are deleted during training. The WeatherGenerator model learns to fill them back in, which requires learning the statistical correlation between the datasets. The results of this learning process can subsequently, for example, be used to fill in missing information if the input streams only cover some data. Masking any future information and trying to predict it enables this architecture to do actual forecasting.
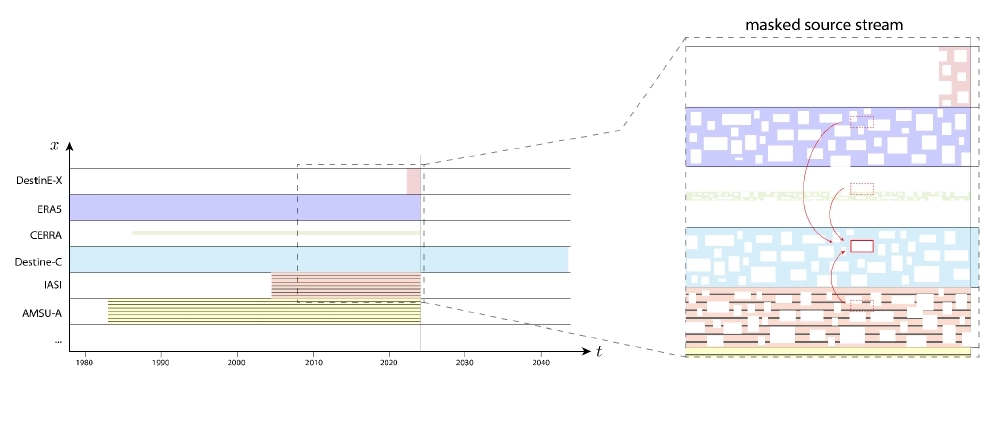
Once fully trained, the network will be able to transform input data into different input streams, and to fill gaps in both space and time.
Weather Generator Applications & Buluttan’s Renewable Energy Expertise
The foundational nature of WeatherGenerator allows any kind of weather intelligence application to be built on top.
Applications will typically work with one of the task-specific decoders and will have a choice to either fine-tune them or extend the decoder with a tail network. Both approaches will have its own trade-offs and the application developers will be researching various approaches to come up with the best way to work with the foundational model.
Buluttan has a portfolio of renewable energy products where state of the art machine learning approaches are being skillfully combined with meteorological LAMs. As part of the WeatherGenerator project, Buluttan will research and develop renewable energy algorithms using the concepts from the generative AI landscape. It will then benchmark the performance to show the impact of the foundational WeatherGenerator model on the renewable energy algorithms’ accuracy.
Next Steps
- The four-year project will have its kick-off meeting in February 2025 with all of its 16 partners.
- The project will organize virtual webinars by both internal and external speakers that will be announced via the project webpage, social media and the project email lists.
- Three external hackathons, two service meetings, and two gender equality meetings will be held to disseminate the ideas and achievements of the project.
Conclusion
Buluttan is eager to push the frontiers of AI-based Weather Forecasting along with European Centre for Medium-Range Weather Forecasts (ECMWF) towards creating a novel and more accurate forecasting approach. Looking forward to collaborating with our European partners on this AI driven journey.
The recently confirmed EU-Horizon project, named WeatherGenerator, aims to build a Generative AI inspired foundational model that can be used & adapted for tasks such as weather forecasting, data assimilation, post-processing and many more.
We, as Buluttan, will utilize our renewable energy forecasting expertise and research how we can take our existing algorithms & products to the next level via WeatherGenerator. We will benchmark the performance and show how much financial gain (€) we will be providing to our existing & new customers. Looking forward to setting a new standard in renewable energy production forecasting.